Fifth Edition
Interview with Manaswi Mishra
Part I
By Don Franzen and Judith Finell
On July 12, 2023, Your Inside Track‘s co-editors interviewed Manaswi Mishra, a graduate researcher at the MIT Media Lab. They discussed recent developments in Al generative music and their implications for copyright law and the music industry.
Don Franzen
For our readership, it would be helpful to understand better what steps, technologically and scientifically speaking, are there to go from words and music to something that AI is able to process and deal with. Could you please explain the training process that leads up to the ability to have a generative AI creation?
Manaswi Mishra
This is a wonderful place to start because we are asking: what is music? Outside of our perception, how is music represented and in what form it is digitally fixed or notated. Once we have it in a fixed form, that’s when we can present it to our AI and computational systems. This representation form has been changing over time, and it began with – you did make a distinction between music as words and sounds. So we could continue that and say, words can come from all the different AI language models presently that can generate sequences of words, sentences, paragraphs, poems and lyrics that can inspire music. Our use of language models to compose ideas is a whole emerging field unto itself. But specifically music can be represented in various fixed forms, also, in many different hierarchical units. The simplest way of representing music, the oldest, is to write down the score of the music, the notations, exactly how they are intended by the composer, with a few added instructions on how to articulate the notes, how hard or fast to play them.
But when you look at a representation like that, like a score, if you are trained in music, you can auralize what that sounds like. If you are not trained in music, you can’t really understand how it would sound until you hear it performed or played back. Some of the early symbolic AI models actually looked at just that, the score representation of music. So, while presenting just this symbolic music, the AI systems have no access to the information of what instruments played it, how each note was articulated, and the many layers of effects and recording artifacts. So until about 8-10 years ago, we were definitely working just with the symbols of how music is represented and not the sounds themselves.
Judith Finell
So if you were to give it, say, an orchestral score of a Beethoven symphony with all of the individual instrumental lines showing, are you saying that it couldn’t reproduce those as instrumental sounds?
Manaswi Mishra
Let’s say you look at the score and imagine what this sounds like, you probably know what each instrument is supposed to sound like, but in every performance it’s going to be different, right? How loud, the intonations, how old is the instrument, what is the size of the room, maybe even how the particular conductor decides to interpret the score on the day of the performance. There are so many aspects of what the sound will actually sound like that are not represented in just the score. Think about the difference between written text versus how it is sounded out.
Whenever we refer to an AI model in today’s context, it basically means there is some form of pattern recognition from the data that is provided to it. AI is a much broader field involving linguistics, philosophy and computation. Symbolic AI systems learn grammar and linguistic rules from the data, but what we are now talking about is neural networks, a specific class of AI that looks at a bunch of data and then learns non-linear patterns within it. So the first question is, what are the steps involved in converting a musical work, like the Beethoven Symphony into a format that such an AI system can process? The simplest as we discussed, is just a score. That was the first thing that was available to the early computer music AI systems1.
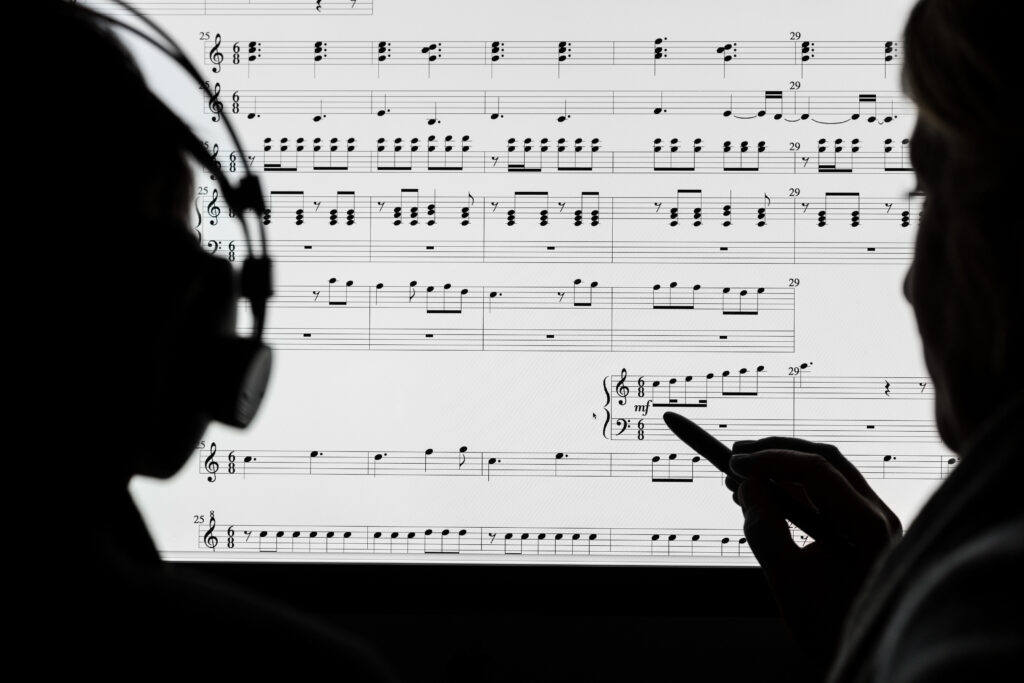
In this early method, the focus was on generating musically sound sequences of notes but every note was played at the same fixed loudness and timing, without expression. The works that are produced copy those patterns of sequences, but they don’t have access to information on expressivity, small timing differences between each note and things like that. Fast forward to the last five years, when we have models that we can now train with direct sound as data. We are talking about how music is represented – We just looked at representing music as a score on a piece of paper, but it can also be represented in all its detail as the sound waves themselves, may be fixed on a tape machine, now recorded digitally at a certain sampling rate in these file formats / CD quality. This is how we commonly represent music today – in a world of streaming high-quality music.
We’re at the early stages of this new period of working with the raw audio directly. There are some challenges to this. First of all, there are patterns in music that happen over a few seconds, like the previous note and the next note, with context, perhaps it’s like a descending melody line. And therefore the note now is related to the previous notes. But there are also patterns that happen over minutes because a chorus might reappear that happened like a minute ago. So you want to be able to learn short and long-term representations. The AI has to model representations in time that are over a few seconds to over a few minutes, maybe if it’s an even larger piece it is something that happens over an entire hour. And this is a major challenge because when we were representing music as symbols, maybe we had 500 or 1,000 symbols/notes for a piece, but when we represent it as raw audio, which is a pressure wave, a vibration, it is sampled at a frequency that is how often you make this measurement of the sound pressure wave (which is how a microphone records). So if it is sampled at 44 kilohertz, that is 44,000 numbers captured every second representing the music. And so if your AI model has to understand something that happened a minute ago, that means it has to look back at samples that are 44,000 samples every second, times 60 seconds back. That’s a long sequence of data. Compare this to the best current language models that are able to learn patterns across a few 1000s of words only. So it was a challenge to make these models practically.
Over the last couple of years, we have started making better and better advancements at being able to model things that happened over more complicated and longer sequences. The first way to address this challenge was just to reduce the sampling rate and just resample the music at only 8,000 Hertz or 16,000 Hertz. So we’ll have less data to work with, a smaller time series to represent one minute of audio, but present innovative methods allow us to do it at 44 kilohertz (CD quality) as well. Yet we are only able to model short, small snippets of ideas. But we’re still at the beginning of this technology, so we are not yet able to take a ten-minute-long piece and then extend it to hours. But to your question, we are able to feed or ingest into our AI models pure sound, and in this sound there are many things that weren’t represented in just symbolic music earlier, like the tones, the timing information, the expressivity, the acoustics of the room, the way it was recorded, the effects added in mixing and mastering post-production.
I can share some more context of the various ways in which music is represented computationally from symbols to raw audio2.
Click to read Part II of this interview
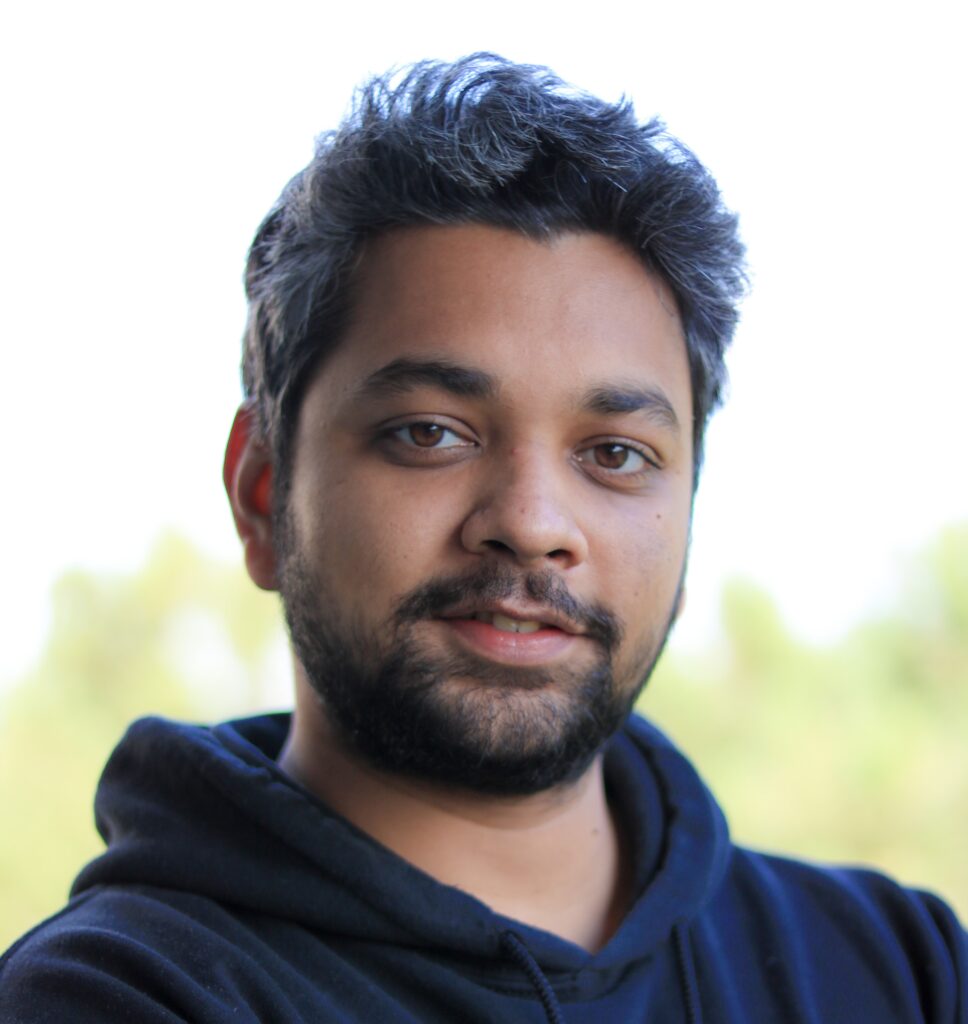
Manaswi Mishra is a current graduate researcher and LEGO Pappert fellow at the Opera of the
Future research group, MIT Media Labs. His research explores strategies and frameworks for a
new age of composing, performing, and learning music using AI. He joined the MIT Media Lab
in 2019 and completed his MS in Media Arts and Science, developing his work “Living, Singing
AI,” to democratize the potential of AI music making with just the human voice. Prior to joining
MIT, he has received a master’s in Music Technology at UPF, Barcelona and bachelor’s in
Technology at the Indian Institute of Technology Madras. He is passionate about a creative future
where every individual can express, reflect, create, and connect through music. Manaswi is also a
founding instigator of the Music Tech Community in India and has organized workshops,
hackathons, and community events to foster a future of music and technology in his home
country.
Footnotes
- Cope, David. “Computer modeling of musical intelligence in EMI.” Computer Music Journal 16.2 (1992): 69-83; Pachet, Francois. “The continuator: Musical interaction with style.” Journal of New Music Research 32.3 (2003): 333-341. ↩︎
- Mishra, Manaswi. MIT Media Lab Thesis: Living, Singing AI, An evolving, intelligent, scalable, bespoke composition system (2019); Dannenberg, Roger B. “Music representation issues, techniques, and systems.” Computer Music Journal 17.3 (1993): 20-30. ↩︎